Abstract: A simple time-dependent model of Earth surface temperatures over the 24 hr day/night cycle at different latitudes is presented. The model reaches energy equilibrium after 1.5 months no matter what temperature it is initialized at. It is shown that even with 1,370 W/m2 of solar flux (reduced by an assumed albedo of 0.3), temperatures at all latitudes remain very cold, even in the afternoon and in the deep tropics. Variation of the model input parameters over reasonable ranges do not change this fact. This demonstrates the importance of the atmospheric “greenhouse” effect, which increases surface temperatures well above what can be achieved with only solar heating and surface infrared loss to outer space.
As a follow-up to yesterday’s post regarding why climate scientists use ~340 W/m2 as the global average solar flux available to the climate system, here I present a model which includes how the incident solar flux (starting with the 1,370 W/m2 solar constant) varies across the Earth as a function of latitude and every 15 minutes throughout the diurnal (day/night) cycle.
I am providing this model to avoid any objections regarding how much solar energy is input into the climate system on average, how that averaging should be done (or whether it is even physically meaningful), whether the nighttime lack of any solar flux should be excluded from the averaging, whether certain assumptions constitute a “flat-Earth” mentality, etc. Instead, the model uses the actual variations of the incident solar radiation on the (assumed spherical) Earth as a function of latitude and time of day. For simplicity, equinox conditions are assumed and so there is no seasonal cycle.
This is not meant to be a realistic model of regional climate; instead, it goes beyond the global averages in the Kiehl-Trenberth energy budget diagram and shows how unrealistically cold temperatures are when you assume there is no greenhouse effect — even in the deep tropics during the afternoon. The model “evolves” the final temperatures, from any starting temperature you specify, based upon a simple energy budget equation (energy conservation) combined with an assumed surface heat capacity. Imbalances between absorbed solar energy and emitted IR energy cause a temperature change which eventually stops (in a long-term average sense) when the daily rate of emitted IR energy equals the daily absorbed solar energy.
The time-dependent model has adjustable inputs: the solar constant (1,370 W/m2); an albedo (for simplicity assumed 0.3 everywhere); the depth of the surface layer responding to solar heating (using the heat capacity of water, but soil heat capacity is similar); and, the assumed broadband infrared emissivity of the surface controlling how fast energy is lost to space as the surface warms. I set the time step to 15 minutes to resolve the diurnal cycle. The Excel model is here, and you are free to change the input parameters and see the results.
Here’s how the incident solar flux changes with time-of-day and latitude. This should not be controversial, since it is just based upon geometry. Even though I only do model calculations at latitudes of 5, 15, 25, 35, 45, 55, 65, 75, and 85 deg. (north and south), the global, 24-hr average incident solar flux is very close to simply 1,370 divided by 4, which is the ratio of the surface areas of a circle and a sphere having the same radius:
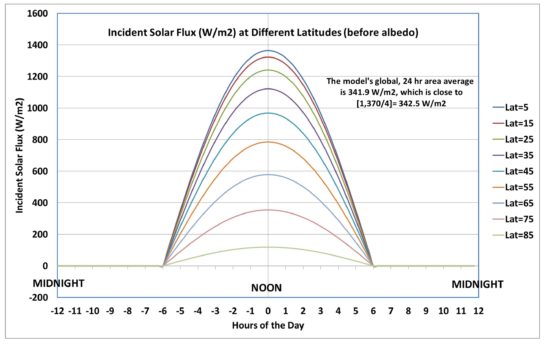
If I had done calculations for every 1 deg. of latitude, the model result would have been exceedingly close to 1,370/4.
If I assume the surface layer responding to heating is 0.1 m deep, a global albedo of 0.3, and a broadband IR emissivity of 0.98, and run the model for 46 days, the model reaches very nearly a steady-state energy equilibrium no matter what temperature I initialize it at (say, 100K or 300 K):
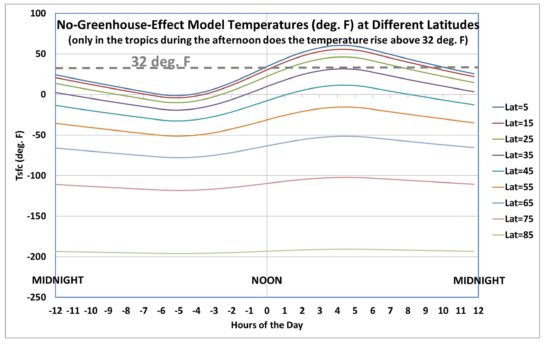
Note that even in the deep tropics, the average temperature is only 29 deg. F. At 45 deg. latitude, the temperature averages -11 deg. F. The diurnal temperature variations are very large, partly because the greenhouse effect in nature helps retain surface energy at night, keeping temperatures from falling too fast like it does in the model.
There is no realistic way to remove the very cold bias of the model without including an atmospheric greenhouse effect. If you object that convection has been ignored, that is a surface cooling (not warming) process, so including convection will only make matters worse. The lack of model heat transport out of the tropics, similarly, would only make the model tropical temperatures colder, not warmer, if it was included. The supposed warming caused by atmospheric pressure that some believe is an alternative theory to the GHE would cause (as Willis Eschenbach has pointed out) surface temperatures to rise, making the surface lose more energy to space than it gains from the sun, and there would no longer be energy balance, violating the 1st Law of Thermodynamics. The temperature would simply go back down again to achieve energy balance (we wouldn’t want to violate the 1st Law).
I hope this will help convince some who are still open-minded on this subject that even intense tropical sunshine cannot explain real-world tropical temperatures. The atmospheric greenhouse effect must also be included. The temperature (of anything) is not determined by the rate of energy input (say, the intensity of sunlight, or how fast your car engine burns gas); it is the result of a balance between energy gain and energy loss. The greenhouse effect reduces the rate of energy loss at the surface, thus causing higher temperatures then if it did not exist.